张忠元教授及其博士生团队在我校AAA级期刊Information Sciences发表了桥接联邦聚类和深度生成模型的论文。
传统聚类方法通常假设数据可以集中到中央服务器上进行处理,但在许多实际应用中,数据分布在多个独立的客户端,隐私保护要求限制了这些本地数据的共享与集中处理。因此,联邦聚类应运而生,使多个客户端能够在不共享原始数据的情况下协同完成数据分组任务。现有的联邦聚类方法通常是传统聚类方法的拓展,例如拓展于k-means(KM)和fuzzy c-means (FCM)的k-FED和FFCM。然而,客户端之间的数据分布往往并不是独立同分布的,这会导致模型性能欠佳。为解决这一问题,该论文将联邦聚类和深度生成模型相结合,通过在合成数据上应用KM或者FCM,模型可以免受非独立同分布问题的影响。此外,受益于合成数据,该模型在客户端和中央服务器之间只需要一轮通讯、鲁棒于设备失联、无需共享原始数据,从而有效地缓解了高通讯成本、系统异质性和隐私保护等核心挑战。下载链接 https://authors.elsevier.com/a/1jUSm4ZQEFi2c
论文题目:SDA-FC: Bridging federated clustering and deep generative model
论文摘要: Federated clustering (FC) is an extension of centralized clustering in federated settings. The key here is how to construct a global similarity measure without sharing private data, since the local similarity may be insufficient to group local data correctly, and the similarity of samples across clients cannot be directly measured due to privacy constraints. Obviously, the most straightforward way to analyze FC is to employ methods extended from centralized ones, such as K-means (KM) and fuzzy c-means (FCM). However, they are vulnerable to non independent-andidentically-distributed (non-IID) data among clients. To handle this, we propose a pretty simple and effective federated clustering framework instantiated with generative adversarial network (GAN), named synthetic data aided federated clustering (SDA-FC). It trains generative adversarial network locally in each client and uploads the generated synthetic data to the server, where KM or FCM is performed on the synthetic data. The synthetic data can make the model immune to the non-IID problem and enable us to capture the global similarity characteristics more effectively without sharing private data. Comprehensive experiments reveal the advantages of SDA-FC, including superior performance in addressing the non-IID problem and the device failures. The code is available at https://github.com/Jarvisyan/SDA-FC.
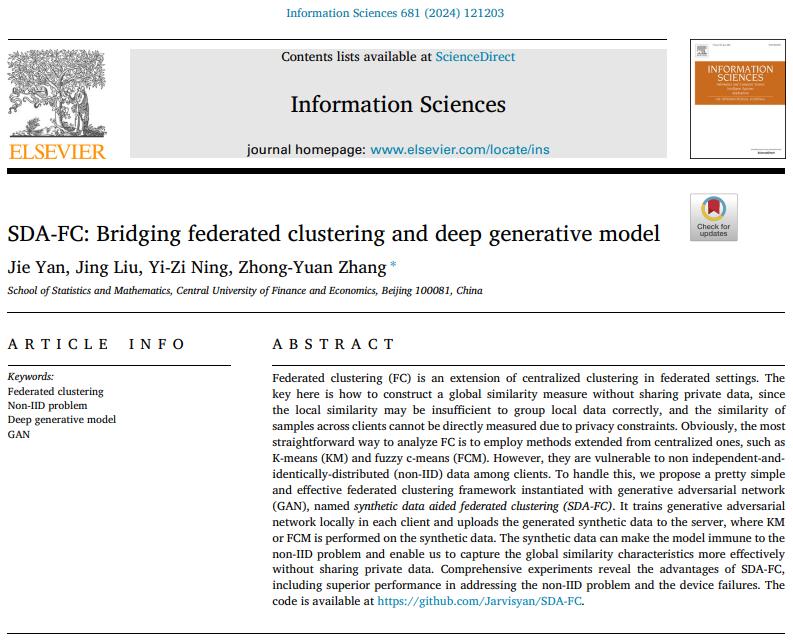
撰稿人:张忠元
审稿人:邓 露