Course outline
Many modern datasets contain a large number of variables, that is they are high dimensional. Such high dimensional datasets are often however characterised by an underlying low dimensional structure. Recovering this low dimensional structure can enable exploratory analysis, visualisation and feature construction. This course takes a journey through the development of dimensionality reduction techniques. They range from linear methods such as Principal Components Analysis and Classical Multidimensional Scaling through to modern Manifold Learning techniques includingIsomap,Laplacian Eigenmaps and Local Linear Embedding. Also covered will be the evaluation of different dimension reduction techniques compared to one another, both with respect to computational considerations and with respect to the fidelity with which they capture the structure of high dimensional data. Applications of dimensionality data to real data problems are also a integral part of this course.
Lecturer
Anastasios Panagiotelis is an Associate Professor of Business Analytics at the University of Sydney Business School. He is also a Director of the International Institute of Forecasters. His work lies in the intersection of business analytics, statistics and econometrics. He conducts research on the development of novel statistical methodology and its application to large scale datasets in energy, macroeconomics and online retail. He has published in a diverse range of top-tier journals including theJournal of the American Statistical Association,Journal of Econometrics,European Journal of Operational Research,Journal of Business and Economic Statistics,Journal of Computational and Graphical StatisticsandInsurance: Mathematics and Economics.
Prerequisites
This course is suitable for students who are interesting in data science and machine learning and have a strong background in statistics. Although the methods covered can be applied to many different datasets, the examples will focus on problems in economics and business. Some basic proficiency in R will be helpful but is not mandatory.
Register the course
Please scan this QR code to register. Registration is open till Nov 14, 24:00.
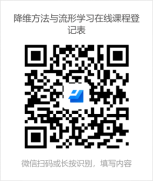
本课程受中央财经大学引智项目支持。
Schedule and contents
Lecture |
Nov 16 Wednesday(Beijing time) |
Topic: Introduction and Classical methods |
9:30-11:00 Module 1: What is High Dimensional Data Analysis? |
1 |
What is High Dimensional Data? |
2 |
Principal Components Analysis |
11:10-12:40 Module 2 :Multidimensional Scaling |
3 |
Multidimensional Scaling |
Lab |
Principal Components and Multidimensional Scaling |
Lecture |
Nov 23 Wednesday(Beijing time) |
Topic: Non-linear dimension reduction |
9:30-11:00 Module 1: Kernel PCA |
1 |
Feature Mappings |
2 |
Kernel PCA |
11:10-12:40 Module 2 : |
3 |
Autoencoders |
Lab |
PCA v Kernel PCA |
Lecture |
Nov 30 Wednesday(Beijing time) |
Topic: Manifold Learning I |
9:30-11:00 Module 1: Manifolds |
1 |
Definitions of manifolds |
2 |
Geometric properties of manifolds |
11:10-12:40 Module 2 :ISOMAP |
3 |
ISOMAP |
Lab |
ISOMAP |
Lecture |
Dec 7 Wednesday(Beijing time) |
Topic: Manifold Learning II |
9:30-11:00 Module 1:More manifold learning algorithms |
1 |
Local linear embedding |
2 |
Laplacian Eigenmaps |
11:10-12:40 Module 2 :Evaluation of manifold learning |
1 |
Quality metrics |
Lab |
Comparison of manifold learning techniques with example |