我院杨玥含教授团队的本科生赵子萱、专硕张雨绮以及学硕禹舜各自在计算机领域顶级期刊 Pattern Recognition、Information Science 以及生统顶级期刊Statistical Methods in Medical Research 发表了关于分类数据、迁移学习以及非稀疏回归问题的研究论文。
其中应用统计专硕张雨绮在杨玥含教授指导下,在我校的3A期刊,同时也是计算机领域顶级期刊 Pattern Recognition 发表了一篇关于迁移学习问题的论文。这篇论文提出了一种称为多源高斯图形模型联合估计(JEM-GGM)的方法,以实现对目标图的稳定和准确的估计。利用来自辅助图的信息,使用所提出的方法有效地解决了样本量小的问题。在该方法中,针对图开发了等价回归模型,并对辅助图和目标图之间的差异进行了惩罚,以确保计算效率并提高估计精度。仿真表明,所提出的方法在估计和预测精度方面总是优于其他方法。将该方法应用于乳腺癌和淋巴癌细胞系揭示了所提出的方法总是获得重要基因组对的稀疏集合。
论文题目:Joint estimation for multisource Gaussian graphical models based on transfer learning
论文摘要:This study considers data from multiple sources for Gaussian graphical models with one target graph and several auxiliary graphs. We propose a method called joint estimation for multisource Gaussian graphical models (JEM-GGM) to achieve a stable and accurate estimate of the target graph. Using the information from the auxiliary graphs, the proposed method is used to effectively solve the problem of small sample sizes. In this method, equivalent regression models are developed for graphs and the difference between the auxiliary and target graphs was penalized to ensure computational efficiency and improve estimation accuracy. Simulations revealed that the proposed method always outperformed other methods in terms of estimation and prediction accuracy. The application of this method to breast and lymphatic cancer cell lines reveals that the proposed method always obtains a sparse collection of important genome pairs.
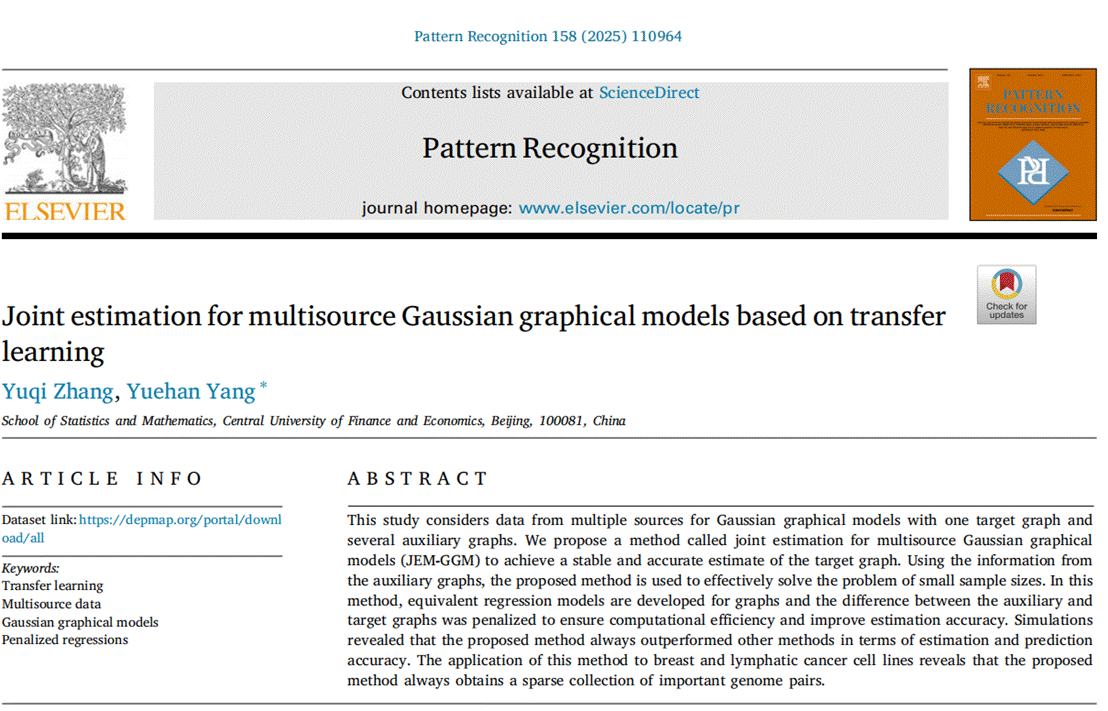
我院概率论与数理统计方向学硕禹舜在杨玥含教授的指导下,在我校3A期刊同时也是生统顶级期刊 Statistical Methods in Medical Research 发表了一篇关于分类数据的论文。在本文中,我们专注于估计具有非稀疏结构的数据的建模问题,特别关注表现出高度相关特征的生物数据。生物和金融等各个领域都面临着非稀疏估计的挑战。我们使用所提出的方法解决问题,称为结构化迭代划分。结构化迭代划分有效地将数据划分为非稀疏和稀疏结构,并消除了众多不相关的变量,在保持计算效率的同时显着降低了误差。数值和理论结果证明了所提出的方法在广泛问题上的竞争优势,并且所提出的方法在与几种现有方法的数值比较中表现出出色的统计性能。我们将所提出的算法应用于两个生物学问题,基因微阵列数据集和嵌合蛋白质数据集,分别用于乳腺癌和阿尔茨海默病远处转移的预后风险。结构化迭代划分为基因识别和选择提供了见解,我们还在预测癌症风险和识别关键因素方面提供了有意义的结果。
论文题目:A structured iterative division approach for non-sparse regression models and
applications in biological data analysis
论文摘要:In this paper, we focus on the modeling problem of estimating data with non-sparse structures, specifically focusing on biological data that exhibit a high degree of relevant features. Various fields, such as biology and finance, face the challenge of non-sparse estimation. We address the problems using the proposed method, called structured iterative division. Structured iterative division effectively divides data into non-sparse and sparse structures and eliminates numerous irrelevant variables, significantly reducing the error while maintaining computational efficiency. Numerical and theoretical results demonstrate the competitive advantage of the proposed method on a wide range of problems, and the proposed method exhibits excellent statistical performance in numerical comparisons with several existing methods. We apply the proposed algorithm to two biology problems, gene microarray datasets, and chimeric protein datasets, to the prognostic risk of distant metastasis in breast cancer and Alzheimer’s disease, respectively. Structured iterative division provides insights into gene identification and selection, and we also provide meaningful results in anticipating cancer risk and identifying key factors.
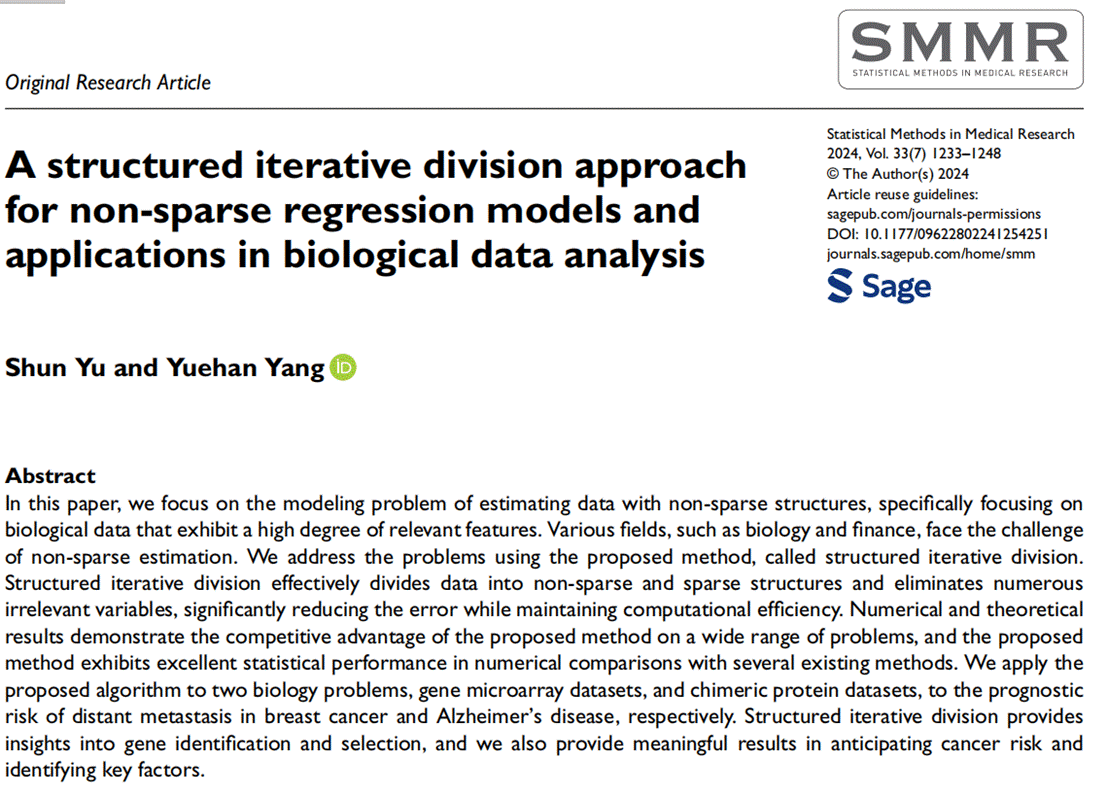
此外,经济统计学专业本科生赵子萱在杨玥含教授指导下,在我校3A期刊同时也是计算机领域顶级期刊 Information Science 发表了一篇关于分类数据的论文。分层分类数据在社会科学、遗传学和其他领域中很常见。分层结构中变量之间的相互作用引入了建模和预测的复杂性。我们专注于对具有分层分类变量的高维线性模型进行建模,并介绍了一种有效的方法。所提出的方法在处理高维分类数据时提供了计算优势。在理论部分,我们证明了解的唯一性,并证明了所提出的估计器在高概率下收敛了最小二乘解。此外,我们展示了我们的方法在两个真实世界数据集上的有效性,一个癌症注册数据集和一个成人数据集,以及模拟数据集,其中我们的方法在预测准确性、变量选择和模型复杂性方面优于比较方法。
论文题目:Nonconvex fusion penalties for high-dimensional hierarchical categorical variables
论文摘要:Hierarchical categorical data is commonly encountered in social science, genetics, and other fields. The interactions between variables in hierarchical structures introduce complexity in
modeling and predicting. We focus on modeling the high-dimensional linear models with hierarchical categorical variables and introduce an efficient method. The proposed method offers
computational advantages when dealing with high-dimensional categorical data. In the theoretical part, we demonstrate the uniqueness of the solution and show that the proposed estimator converges the least square solution under the high probability. Additionally, we showcase the effectiveness of our method on two real-world datasets, a cancer-reg dataset and an adult dataset, and simulated datasets, where our method outperforms comparative approaches in terms of predictive accuracy, variable selection, and model complexity.
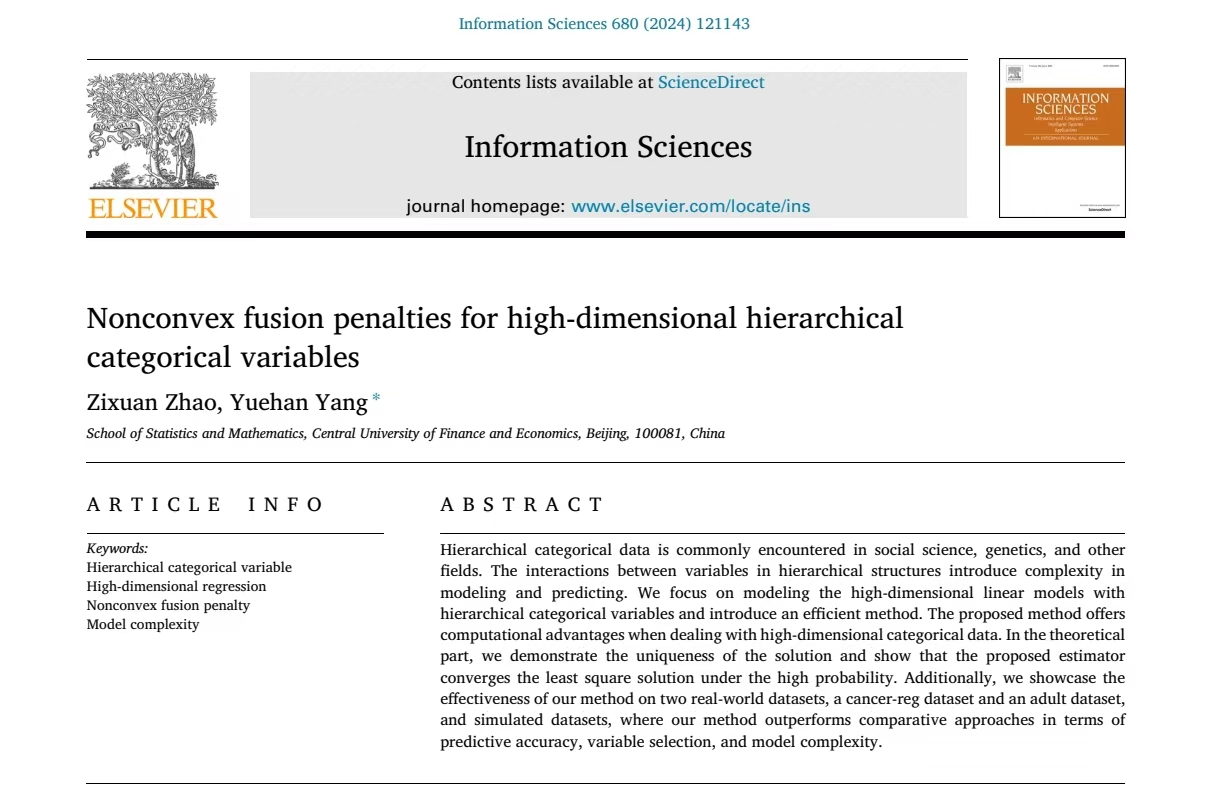
作者介绍:
杨玥含,中央财经大学教授,龙马青年学者,主要从事多重结构数据建模、因果推断、迁移学习等研究,作为独立作者、第一作者或通讯作者在统计学四大期刊 Journal of the American Statistical Association、Biometrika、经济学顶级期刊 Journal of Business and Economics Statistics,人工智能顶级期刊 Pattern Recognition、Knowledge-Based Systems 等期刊发表论文近40篇。
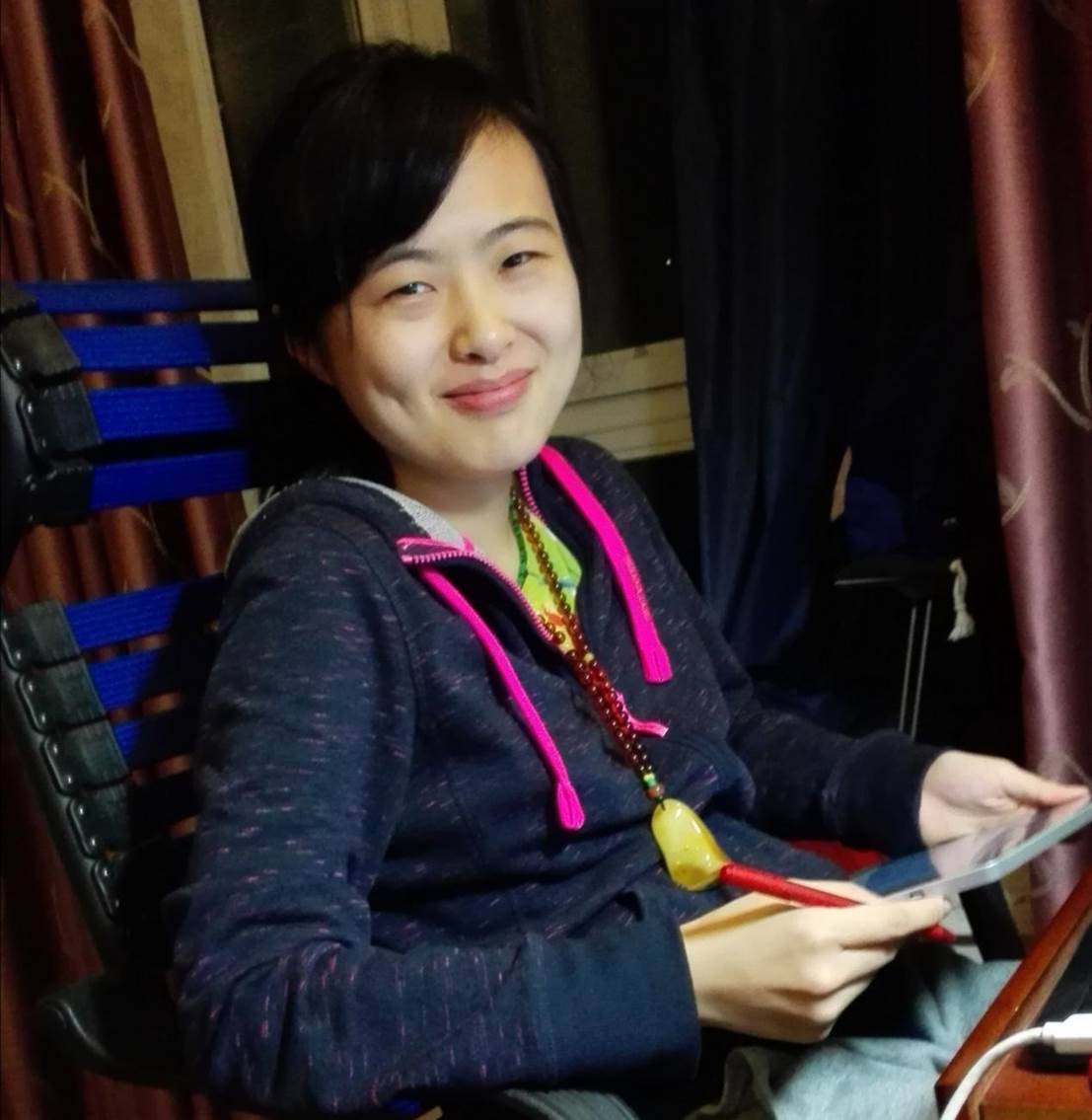
撰稿人:杨玥含
审稿人:邓露